How AI is transforming predictive analytics in business
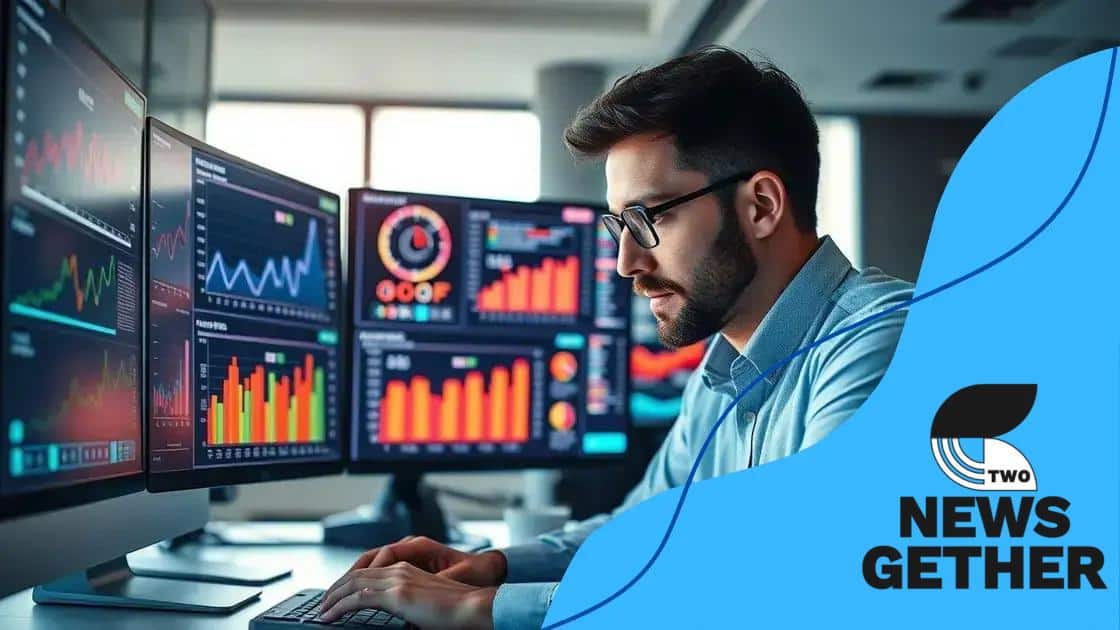
AI is transforming predictive analytics in business by enabling real-time data analysis, automating processes, and improving customer insights, which enhances decision-making and operational efficiency.
How AI is transforming predictive analytics in business is a topic that’s gaining traction. With data at our fingertips, businesses now have the ability to predict trends and consumer behavior like never before. So, what does this mean for your organization?
Understanding predictive analytics in the age of AI
In today’s business landscape, understanding predictive analytics in the age of AI is crucial. Companies can harness the power of data to make informed decisions and forecast trends. With AI technologies, organizations can analyze patterns in consumer behavior and market conditions, giving them a competitive edge.
What Is Predictive Analytics?
Predictive analytics involves using historical data, statistical algorithms, and machine learning techniques to identify the likelihood of future outcomes. This approach allows businesses to anticipate customer needs, forecast sales, and optimize operations.
Key Components of Predictive Analytics
- Data Collection: Gathering relevant data from various sources.
- Statistical Analysis: Utilizing algorithms to analyze data and find trends.
- Modeling: Creating models that represent potential future outcomes.
- Validation: Testing models against new data to ensure accuracy.
As organizations integrate AI into their analytics, they can improve the accuracy of their predictions. For example, businesses leveraging machine learning can adjust their models based on new data inputs, leading to more precise forecasting.
Moreover, developing a strong understanding of predictive analytics allows companies to enhance customer experiences. By anticipating what customers want, they can tailor their services and products accordingly.
Challenges in Predictive Analytics
While there are many benefits, companies face challenges such as data quality and integration. Poor data can lead to inaccurate predictions. Therefore, it’s essential to ensure that the data used is reliable and relevant.
Additionally, businesses must focus on training their teams to utilize predictive analytics effectively. Without proper understanding, the potential of AI can go untapped.
Key benefits of AI-driven predictive analytics
AI-driven predictive analytics offers several advantages that can reshape business strategies. Companies can leverage their data more effectively, gaining insights that enhance decision-making processes. One significant benefit is improved accuracy in forecasting. With advanced algorithms, businesses can analyze vast datasets, leading to more precise predictions.
Enhanced Efficiency
By automating data analysis, organizations can save time and resources. AI reduces the need for manual data sifting, allowing teams to focus on strategic initiatives. This not only streamlines operations but also accelerates the decision-making process.
Better Customer Insights
AI can identify patterns in customer behavior, helping businesses tailor their offerings. Understanding what customers want leads to improved customer satisfaction and retention. Companies can use these insights to develop targeted marketing strategies, maximizing their impact.
- Increased personalization of marketing efforts.
- Predictive models help anticipate customer needs.
- Enhanced loyalty through tailored experiences.
Another key advantage is risk management. By predicting potential challenges and trends, organizations can mitigate risks before they escalate. This proactive approach helps maintain stability, especially in unpredictable markets.
Additionally, AI-driven analytics aids in resource allocation. Businesses can identify areas where resources should be focused to maximize returns. This approach not only improves operational efficiency but also drives innovation, fostering a culture of continuous improvement.
Real-world applications of AI in business analytics
Real-world applications of AI in business analytics are reshaping how organizations operate. Many companies are now using AI tools to enhance their decision-making processes. This technology enables businesses to analyze vast amounts of data quickly and accurately, leading to valuable insights.
Customer Service Improvement
AI is revolutionizing customer service through chatbots and virtual assistants. These tools can handle customer inquiries 24/7, providing immediate responses. This availability improves customer experience and satisfaction. Additionally, AI analyzes customer interactions to enhance service quality over time.
Marketing Personalization
Businesses are using AI to tailor marketing strategies to individual preferences. By analyzing purchasing behavior and online activity, companies create personalized recommendations. This targeted approach increases customer engagement and conversion rates.
- Segmentation of customers based on behavior.
- Automated email campaigns based on user preferences.
- Customized product suggestions in real-time.
Moreover, AI-powered analytics are helping organizations optimize their supply chains. By predicting demand fluctuations, businesses can adjust inventory levels accordingly. This reduces excess stock and ensures that products are available when customers need them. By doing so, companies can enhance operational efficiency.
AI also plays a vital role in financial forecasting. Organizations utilize predictive analytics to project future sales and expenses. This capability allows businesses to allocate resources more effectively and plan for growth. Utilizing AI in such ways fosters a proactive approach to financial management.
Challenges in integrating AI with predictive models
The process of integrating AI with predictive models presents several challenges that businesses must navigate. One of the primary difficulties is ensuring data quality. Inaccurate or incomplete data can lead to erroneous predictions, undermining the entire predictive analytics effort. Organizations need to invest in data cleaning and validation tools to maintain high standards.
Technical Complexity
Integrating AI systems with existing predictive models can also be technically complex. Different systems may use various formats and standards, complicating the data integration process. These technical hurdles require skilled personnel who understand both AI and the existing systems.
Change Management
Another challenge is change management within organizations. Employees may resist adopting new AI-driven tools, fearing job changes or skill obsolescence. To overcome this, companies should prioritize training and emphasize the benefits of AI integration, fostering a culture of innovation.
- Clear communication about roles and benefits.
- Providing adequate training sessions.
- Involving employees in the integration process.
Additionally, the ethical implications of AI must be considered. Organizations need to ensure that their use of AI aligns with ethical standards and regulations. This can lead to additional compliance challenges, especially for industries with strict guidelines.
Furthermore, ongoing maintenance and updates are crucial. AI models require regular adjustments to remain effective as new data becomes available. Companies must establish protocols to ensure that their models adapt to changing environments and data landscapes. This ongoing commitment can be resource-intensive but is essential for success.
Future trends in AI and predictive analytics
Future trends in AI and predictive analytics promise to revolutionize how businesses operate. As technology evolves, we can expect more sophisticated algorithms that enhance predictive capabilities. One significant trend is the growth of real-time data analysis. Businesses will increasingly rely on immediate insights to make quick decisions, adapting rapidly to changing conditions.
Integration of AI with Internet of Things (IoT)
The integration of AI with IoT devices is set to reshape predictive analytics. Smart devices will collect vast amounts of data, which AI can analyze to forecast trends and behaviors. This combination will allow companies to improve operations and create personalized customer experiences.
Enhanced Automation
Automation of data processes will continue to advance. AI systems will handle high volumes of data without human intervention, reducing errors and increasing efficiency. This trend will free up employees to focus on strategic tasks rather than mundane data management.
- Streamlined operations through automated systems.
- Reduced time in data processing and analysis.
- Enhanced accuracy in predictions.
Another trend is the focus on ethical AI practices. As AI becomes more prevalent, the importance of using ethical guidelines in predictive analytics will rise. Companies will need to address issues like data privacy and algorithm bias to maintain consumer trust.
Additionally, the rise of democratized AI tools will allow more businesses to access advanced analytics. These tools will be user-friendly, allowing employees from various backgrounds to leverage AI insights without needing extensive technical training. This shift could lead to increased innovation across industries.
FAQ – Frequently Asked Questions about AI and Predictive Analytics
What is predictive analytics?
Predictive analytics uses historical data and AI algorithms to forecast future outcomes, helping businesses make informed decisions.
How can AI enhance customer experience?
AI analyzes customer behavior to provide personalized recommendations and immediate support through tools like chatbots, improving overall satisfaction.
What challenges might companies face with AI integration?
Companies may encounter data quality issues, technical complexity, and resistance from employees when adopting AI-driven solutions.
Why is ethical AI important?
Ethical AI ensures that data privacy is respected and that algorithms do not perpetuate bias, maintaining consumer trust in AI technologies.